Quantum-Enhanced Machine Learning with Classiq
Revolutionizing AI with Quantum Computing
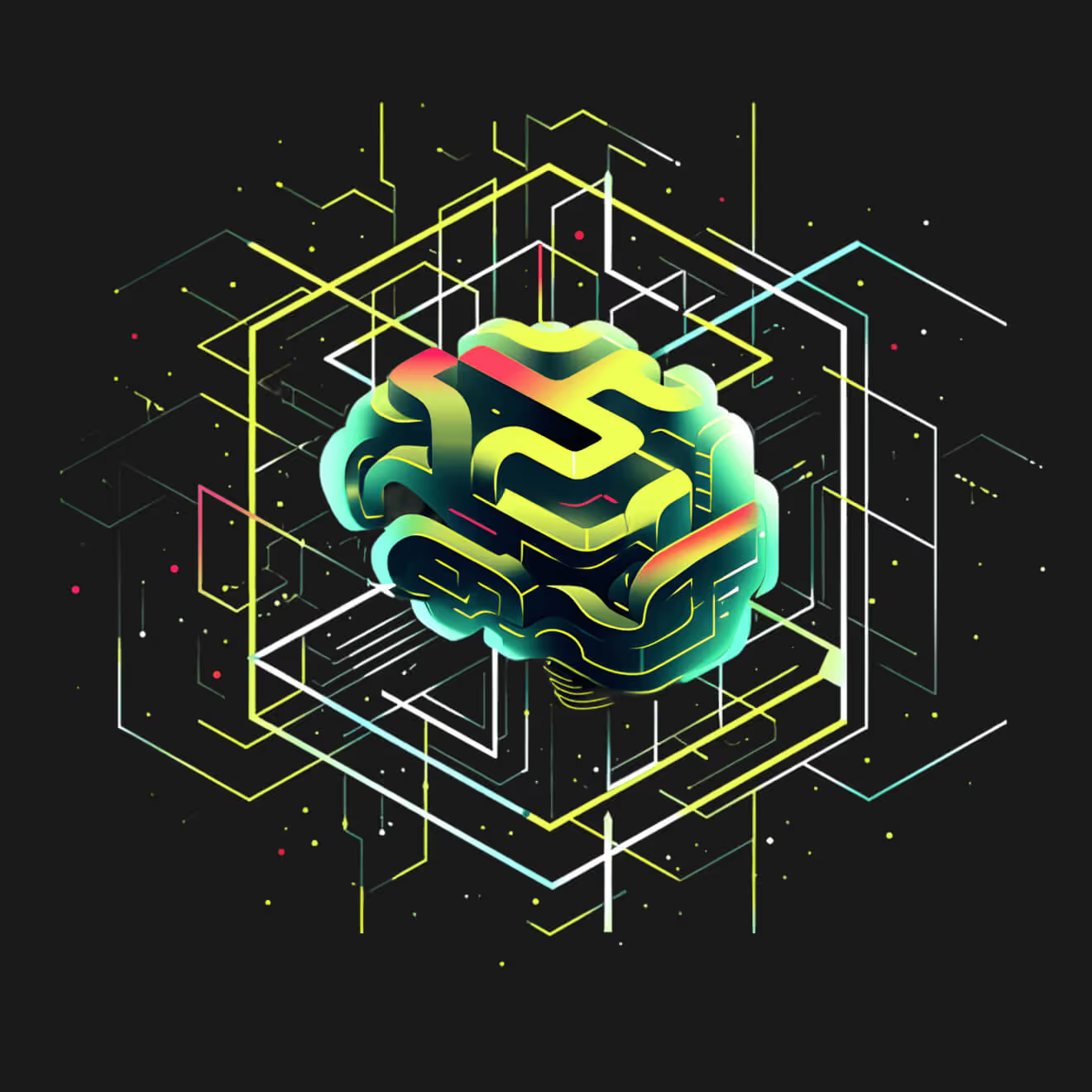
Harnessing Quantum Computing in Machine Learning
Machine learning, integral to modern AI, is poised for a quantum leap with quantum computing's capabilities in parallel processing and probabilistic algorithms. Classical machine learning, often constrained by the sheer volume of data and complexity of computations, finds a powerful ally in quantum computing. This new approach dramatically enhances the processing and analysis of large datasets, optimizing complex algorithms with an efficiency beyond the reach of classical computing. For instance, in tasks like neural network training or pattern recognition, quantum machine learning algorithms can significantly accelerate performance, pushing the boundaries of AI development.Classiq’s platform democratizes access to these quantum advancements. It enables users to formulate machine learning tasks in a quantum-compatible framework, transforming them into optimized quantum circuits. These circuits are meticulously designed to harness the strengths of quantum processors, offering a tailored approach to advanced AI applications. From rapid, comprehensive data analysis to the development of sophisticated, real-time decision-making algorithms, Classiq's platform is at the forefront, ushering in a new era of AI innovation powered by quantum computing.
Key Quantum Algorithms for Machine Learning on Classiq
.jpg)
Key Quantum Algorithms for Machine Learning on Classiq
Several quantum algorithms are particularly suited for machine learning tasks on Classiq's platform:
Integrates quantum computing principles into neural networks, enhancing tasks like pattern recognition and complex decision making.
QSVM offers efficient data classification, making it suitable for categorizing and retrieving specific data in extensive database systems.
VQE is a hybrid quantum-classical algorithm designed to find the lowest eigenvalue of a Hamiltonian (energy function), making it highly suitable for complex optimization tasks, including knapsack problems. It iteratively adjusts quantum circuits to approach the optimal solution.
An algorithm that uses quantum mechanics to approximate solutions for combinatorial optimization problems like knapsack issues. QAOA balances between performance and resource use, finding near-optimal solutions with high efficiency, especially in scenarios with multiple constraints.
A quantum search algorithm that significantly accelerates the process of finding a specific item within an unsorted database. For knapsack problems, it provides a quadratic speedup in identifying optimal solutions, making it highly efficient for large datasets.