Is Quantum Computing the Key to Unlocking the Future of Electric Vehicles and Battery Innovation?
Electric Dreams and Quantum Leaps: How Quantum Computing Can Revolutionize EVs
In the realm of transportation, electric vehicles (EVs) are rapidly gaining prominence as a cleaner and more sustainable alternative to traditional gasoline-powered cars. Governments and automakers worldwide are investing heavily in EV technologies to combat climate change and reduce dependence on fossil fuels. However, widespread adoption of EVs faces significant challenges, particularly in terms of battery performance, range, and cost. Overcoming these hurdles requires accelerating the pace of innovation in battery chemistry and materials science. In recent years, quantum computing has emerged as a potentially game-changing technology that could revolutionize the design and optimization of EV batteries. Just as the principles of quantum mechanics have transformed our understanding of the subatomic world, quantum computers harness these phenomena to perform complex calculations that are intractable for classical computers. These powerful machines could open up new frontiers in simulating and analyzing the behavior of materials and chemical reactions at the molecular level.
The Limitations of Classical Computing: Why Traditional Methods Fall Short in Battery Research
Current research on electric vehicle batteries relies heavily on classical computational methods, such as density functional theory (DFT) calculations, to model the behavior of materials and predict their properties. While these techniques have led to significant advances, they face inherent limitations in accuracy and scalability. Classical simulations often involve approximations and trade-offs between the level of detail captured and the computational complexity involved. For example, accurately modeling the quantum mechanical interactions between electrons in complex materials requires prohibitively large amounts of computing power and time. Consequently, researchers are forced to make simplifications and use coarser-grained models, which can compromise the predictive power of the simulations. Moreover, the combinatorial nature of materials discovery means that even with high-performance computing clusters, it is impractical to exhaustively explore the vast design space of potential battery chemistries and structures. These bottlenecks in computational materials science slow down the pace of innovation and limit the ability to identify optimal solutions for EV batteries.
Quantum Chemistry on Steroids: Unleashing the Power of Qubits for Battery Simulations
Quantum computing offers a paradigm shift in our ability to simulate and understand complex chemical systems, such as those found in electric vehicle batteries. By harnessing the principles of quantum mechanics, quantum computers can efficiently model the behavior of electrons and atoms, enabling ab-initio quantum chemical simulations with unprecedented accuracy. Unlike classical computers, which rely on bits that can only be in a state of 0 or 1, quantum computers use qubits that can exist in multiple states simultaneously, a property known as superposition. This allows quantum computers to perform certain calculations substantually faster than their classical counterparts - very often quadratically oe exponentially. In the context of battery simulations, quantum computers can model the electronic structure of materials with much higher fidelity, reducing the need for approximations and trade-offs. For example, quantum algorithms like the Variational Quantum Eigensolver (VQE) and the Quantum Phase Estimation (QPE) can efficiently solve the Schrödinger equation for complex molecules, enabling the prediction of properties such as redox potentials, ionic conductivities, and reaction energetics with chemical accuracy. This could accelerate the discovery of new electrolyte materials with improved stability, conductivity, and compatibility with electrode materials. Quantum computers can also simulate the intricate interactions and reactions occurring at the interfaces between the electrolyte and the electrodes, which are crucial for understanding phenomena like charge transfer, passivation, and dendrite formation. By accurately modeling the behavior of key components such as ethylene carbonate (solvent), LiPF6 (conducting salt), and fluoroethylene carbonate (additive) at the molecular level, quantum simulations can guide the optimization of electrolyte formulations for enhanced battery performance and safety. Furthermore, quantum computers can potentially simulate fluid dynamics within battery cells at the atomic scale, providing insights into ion diffusion, heat transfer, and mass transport limitations. These capabilities could lead to the design of novel battery architectures with improved power density, faster charging rates, and longer cycle life.
Quantum-Charged Calculations: A Deep Dive into Redox Potential Predictions
To illustrate the potential of quantum computing for battery simulations, let's consider a specific example of calculating the redox potential of a lithium-ion battery electrolyte molecule, such as ethylene carbonate (EC). The redox potential is a crucial property that determines the stability and electrochemical window of the electrolyte, and its accurate prediction is essential for designing high-performance batteries.
In a quantum chemical simulation, the redox potential can be calculated from the difference in the ground-state energies of the neutral and reduced (or oxidized) forms of the molecule. The ground-state energy is obtained by solving the electronic Schrödinger equation:
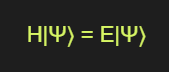
where H is the molecular Hamiltonian operator, |Ψ⟩ is the electronic wavefunction, and E is the energy eigenvalue.
On a quantum computer, the molecular Hamiltonian can be mapped onto a system of qubits using encoding schemes like the Jordan-Wigner or Bravyi-Kitaev transformations. For example, using the Jordan-Wigner encoding, the second-quantized fermionic Hamiltonian of EC (C3H4O3) with 32 electrons and 68 spin-orbitals can be represented as:
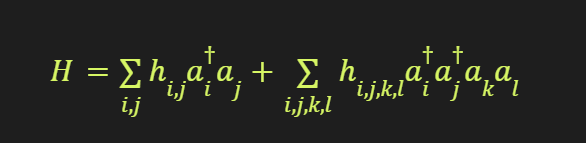
where aᵢ† and aᵢ are the fermionic creation and annihilation operators, and hᵢⱼ and hᵢⱼₖₗ are the one- and two-electron integrals, respectively.
The ground-state energy can then be calculated using the Variational Quantum Eigensolver (VQE) algorithm, which involves preparing a parameterized trial wavefunction |Ψ(θ)⟩ on the quantum computer and minimizing the expectation value of the Hamiltonian:

The trial wavefunction is typically constructed using a quantum circuit with parametrized gates, such as single-qubit rotations and two-qubit entangling gates. The parameters θ are optimized using a classical optimization algorithm, such as gradient descent or the Nelder-Mead method.
For example, a simple VQE circuit for EC might consist of a series of single-qubit Ry rotations and two-qubit CNOT gates:
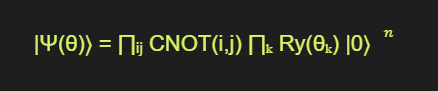
where n is the number of qubits, and the indices i, j, and k represent the qubits involved in each gate.
By evaluating the expectation value of the Hamiltonian on the quantum computer for different values of θ and using a classical optimizer to minimize the energy, the ground-state energy E₀ of the neutral EC molecule can be obtained. The same procedure can be repeated for the reduced (or oxidized) form of the molecule, yielding the energy E₁.
The redox potential (in volts) can then be calculated as:

where n_e is the number of electrons transferred (1 for reduction, -1 for oxidation), and F is the Faraday constant (96,485 C/mol).
For example, if the VQE calculations yield ground-state energies of E₀ = -1,000 Hartree for the neutral EC and E₁ = -1,001 Hartree for the reduced EC, the redox potential would be:

This example demonstrates how quantum computers can be used to calculate important battery properties like redox potentials with high accuracy, guiding the design and optimization of electrolyte materials. As quantum hardware and algorithms continue to improve, these simulations will become increasingly powerful tools for accelerating battery innovation.
Classiq's Quantum Software Platform for Electric Vehicle Applications
Classiq, a leading quantum software company, is developing powerful tools to accelerate the application of quantum computing in the electric vehicle industry. Our platform enables researchers and engineers to design and optimize quantum circuits for complex computational tasks, such as simulating battery materials and training machine learning models, without requiring deep expertise in quantum physics or low-level gate-based programming.
One of the key features of Classiq's software is its ability to automatically generate efficient quantum circuits from high-level functional models. By abstracting away the intricacies of quantum hardware and focusing on the desired computational outcomes, our platform allows users to easily build models of complex quantum algorithms and optimize them for specific hardware. This is particularly relevant for battery simulations, where the choice of quantum encoding, ansatz, and measurement scheme can greatly impact the accuracy and resource requirements of the algorithm.
For example, Classiq's software can help researchers automate the design quantum circuits for calculating the redox potentials of electrolyte molecules, as described in the previous section. By providing a high-level interface to specify the molecular Hamiltonian, the desired precision, and the available quantum resources, our platform can automatically generate an optimized VQE circuit that minimizes the number of qubits and gates required while maximizing the expected accuracy. This can significantly accelerate the discovery and optimization of new battery materials, as researchers can focus on the chemical insights rather than the low-level quantum implementation.
Moreover, Classiq's software can also facilitate the development of hybrid quantum-classical algorithms for machine learning tasks in electric vehicles. By seamlessly integrating quantum circuits with classical data processing and optimization routines, our platform enables the creation of QML models that can leverage the power of quantum computing within the constraints of current hardware. As quantum computers scale up in size and reliability, Classiq's software will continue to provide a flexible and accessible interface for harnessing their capabilities in the automotive industry.
The Road Ahead: Challenges and Opportunities for Quantum Computing in the EV Industry
While quantum computing offers immense potential for revolutionizing electric vehicle batteries and software, there are still significant challenges that need to be overcome before these benefits can be fully realized. One of the main hurdles is the development of fault-tolerant, large-scale quantum computers that can perform reliable, errorless computations on thousands of qubits. Current quantum hardware is still prone to errors and decoherence, which limit the depth and complexity of the algorithms that can be executed. Achieving fault-tolerance requires the implementation of quantum error correction codes, which themselves consume a large number of physical qubits to encode each logical qubit. Estimates suggest that millions of physical qubits may be necessary to simulate complex battery materials with chemical accuracy.
In the near-term, hybrid quantum-classical algorithms, such as variational quantum eigensolvers (VQE) and quantum approximate optimization algorithms (QAOA), offer a pragmatic approach to leveraging the power of quantum computers within their current limitations. These algorithms employ a quantum processor to execute a parameterized quantum circuit, while a classical optimizer is used to adjust the parameters based on the measurement outcomes. By iterating between the quantum and classical components, hybrid algorithms can find approximate solutions to complex optimization problems, such as molecular ground states or optimal control policies. However, the performance of these algorithms is still limited by the noise and scale of current quantum hardware.
As quantum technologies continue to advance, it is crucial to establish a realistic timeline for when quantum advantage might be achieved in the domain of electric vehicles. In the next 5 years, it is likely that quantum computers will be used primarily for small-scale optimization problems and proof-of-concept demonstrations of QML. Significant progress in battery materials discovery and optimization is expected within a 10-year horizon, as quantum simulators with hundreds of qubits become available. However, fully fault-tolerant quantum computers capable of performing accurate, large-scale simulations of entire battery systems may still be 15-20 years away.
To prepare for this quantum future, automotive companies gradually tart engaging with the quantum ecosystem and building in-house expertise. Partnerships between car manufacturers, battery suppliers, and quantum technology companies will be essential to guide the development of quantum algorithms and hardware tailored to the specific needs of the electric vehicle industry. Equally important is the cultivation of a quantum-ready workforce, with cross-disciplinary skills in chemistry, materials science, computer science, and quantum information theory. By investing in these areas now, the automotive sector can position itself to harness the transformative power of quantum computing as it becomes a practical reality in the coming decades.
Electric Dreams and Quantum Leaps: How Quantum Computing Can Revolutionize EVs
In the realm of transportation, electric vehicles (EVs) are rapidly gaining prominence as a cleaner and more sustainable alternative to traditional gasoline-powered cars. Governments and automakers worldwide are investing heavily in EV technologies to combat climate change and reduce dependence on fossil fuels. However, widespread adoption of EVs faces significant challenges, particularly in terms of battery performance, range, and cost. Overcoming these hurdles requires accelerating the pace of innovation in battery chemistry and materials science. In recent years, quantum computing has emerged as a potentially game-changing technology that could revolutionize the design and optimization of EV batteries. Just as the principles of quantum mechanics have transformed our understanding of the subatomic world, quantum computers harness these phenomena to perform complex calculations that are intractable for classical computers. These powerful machines could open up new frontiers in simulating and analyzing the behavior of materials and chemical reactions at the molecular level.
The Limitations of Classical Computing: Why Traditional Methods Fall Short in Battery Research
Current research on electric vehicle batteries relies heavily on classical computational methods, such as density functional theory (DFT) calculations, to model the behavior of materials and predict their properties. While these techniques have led to significant advances, they face inherent limitations in accuracy and scalability. Classical simulations often involve approximations and trade-offs between the level of detail captured and the computational complexity involved. For example, accurately modeling the quantum mechanical interactions between electrons in complex materials requires prohibitively large amounts of computing power and time. Consequently, researchers are forced to make simplifications and use coarser-grained models, which can compromise the predictive power of the simulations. Moreover, the combinatorial nature of materials discovery means that even with high-performance computing clusters, it is impractical to exhaustively explore the vast design space of potential battery chemistries and structures. These bottlenecks in computational materials science slow down the pace of innovation and limit the ability to identify optimal solutions for EV batteries.
Quantum Chemistry on Steroids: Unleashing the Power of Qubits for Battery Simulations
Quantum computing offers a paradigm shift in our ability to simulate and understand complex chemical systems, such as those found in electric vehicle batteries. By harnessing the principles of quantum mechanics, quantum computers can efficiently model the behavior of electrons and atoms, enabling ab-initio quantum chemical simulations with unprecedented accuracy. Unlike classical computers, which rely on bits that can only be in a state of 0 or 1, quantum computers use qubits that can exist in multiple states simultaneously, a property known as superposition. This allows quantum computers to perform certain calculations substantually faster than their classical counterparts - very often quadratically oe exponentially. In the context of battery simulations, quantum computers can model the electronic structure of materials with much higher fidelity, reducing the need for approximations and trade-offs. For example, quantum algorithms like the Variational Quantum Eigensolver (VQE) and the Quantum Phase Estimation (QPE) can efficiently solve the Schrödinger equation for complex molecules, enabling the prediction of properties such as redox potentials, ionic conductivities, and reaction energetics with chemical accuracy. This could accelerate the discovery of new electrolyte materials with improved stability, conductivity, and compatibility with electrode materials. Quantum computers can also simulate the intricate interactions and reactions occurring at the interfaces between the electrolyte and the electrodes, which are crucial for understanding phenomena like charge transfer, passivation, and dendrite formation. By accurately modeling the behavior of key components such as ethylene carbonate (solvent), LiPF6 (conducting salt), and fluoroethylene carbonate (additive) at the molecular level, quantum simulations can guide the optimization of electrolyte formulations for enhanced battery performance and safety. Furthermore, quantum computers can potentially simulate fluid dynamics within battery cells at the atomic scale, providing insights into ion diffusion, heat transfer, and mass transport limitations. These capabilities could lead to the design of novel battery architectures with improved power density, faster charging rates, and longer cycle life.
Quantum-Charged Calculations: A Deep Dive into Redox Potential Predictions
To illustrate the potential of quantum computing for battery simulations, let's consider a specific example of calculating the redox potential of a lithium-ion battery electrolyte molecule, such as ethylene carbonate (EC). The redox potential is a crucial property that determines the stability and electrochemical window of the electrolyte, and its accurate prediction is essential for designing high-performance batteries.
In a quantum chemical simulation, the redox potential can be calculated from the difference in the ground-state energies of the neutral and reduced (or oxidized) forms of the molecule. The ground-state energy is obtained by solving the electronic Schrödinger equation:
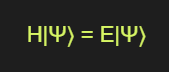
where H is the molecular Hamiltonian operator, |Ψ⟩ is the electronic wavefunction, and E is the energy eigenvalue.
On a quantum computer, the molecular Hamiltonian can be mapped onto a system of qubits using encoding schemes like the Jordan-Wigner or Bravyi-Kitaev transformations. For example, using the Jordan-Wigner encoding, the second-quantized fermionic Hamiltonian of EC (C3H4O3) with 32 electrons and 68 spin-orbitals can be represented as:
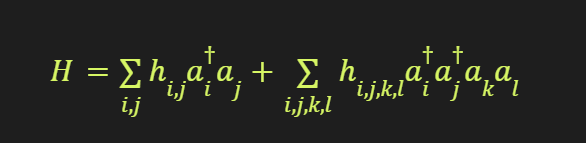
where aᵢ† and aᵢ are the fermionic creation and annihilation operators, and hᵢⱼ and hᵢⱼₖₗ are the one- and two-electron integrals, respectively.
The ground-state energy can then be calculated using the Variational Quantum Eigensolver (VQE) algorithm, which involves preparing a parameterized trial wavefunction |Ψ(θ)⟩ on the quantum computer and minimizing the expectation value of the Hamiltonian:

The trial wavefunction is typically constructed using a quantum circuit with parametrized gates, such as single-qubit rotations and two-qubit entangling gates. The parameters θ are optimized using a classical optimization algorithm, such as gradient descent or the Nelder-Mead method.
For example, a simple VQE circuit for EC might consist of a series of single-qubit Ry rotations and two-qubit CNOT gates:
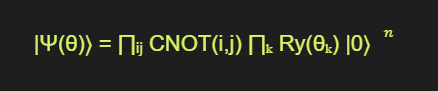
where n is the number of qubits, and the indices i, j, and k represent the qubits involved in each gate.
By evaluating the expectation value of the Hamiltonian on the quantum computer for different values of θ and using a classical optimizer to minimize the energy, the ground-state energy E₀ of the neutral EC molecule can be obtained. The same procedure can be repeated for the reduced (or oxidized) form of the molecule, yielding the energy E₁.
The redox potential (in volts) can then be calculated as:

where n_e is the number of electrons transferred (1 for reduction, -1 for oxidation), and F is the Faraday constant (96,485 C/mol).
For example, if the VQE calculations yield ground-state energies of E₀ = -1,000 Hartree for the neutral EC and E₁ = -1,001 Hartree for the reduced EC, the redox potential would be:

This example demonstrates how quantum computers can be used to calculate important battery properties like redox potentials with high accuracy, guiding the design and optimization of electrolyte materials. As quantum hardware and algorithms continue to improve, these simulations will become increasingly powerful tools for accelerating battery innovation.
Classiq's Quantum Software Platform for Electric Vehicle Applications
Classiq, a leading quantum software company, is developing powerful tools to accelerate the application of quantum computing in the electric vehicle industry. Our platform enables researchers and engineers to design and optimize quantum circuits for complex computational tasks, such as simulating battery materials and training machine learning models, without requiring deep expertise in quantum physics or low-level gate-based programming.
One of the key features of Classiq's software is its ability to automatically generate efficient quantum circuits from high-level functional models. By abstracting away the intricacies of quantum hardware and focusing on the desired computational outcomes, our platform allows users to easily build models of complex quantum algorithms and optimize them for specific hardware. This is particularly relevant for battery simulations, where the choice of quantum encoding, ansatz, and measurement scheme can greatly impact the accuracy and resource requirements of the algorithm.
For example, Classiq's software can help researchers automate the design quantum circuits for calculating the redox potentials of electrolyte molecules, as described in the previous section. By providing a high-level interface to specify the molecular Hamiltonian, the desired precision, and the available quantum resources, our platform can automatically generate an optimized VQE circuit that minimizes the number of qubits and gates required while maximizing the expected accuracy. This can significantly accelerate the discovery and optimization of new battery materials, as researchers can focus on the chemical insights rather than the low-level quantum implementation.
Moreover, Classiq's software can also facilitate the development of hybrid quantum-classical algorithms for machine learning tasks in electric vehicles. By seamlessly integrating quantum circuits with classical data processing and optimization routines, our platform enables the creation of QML models that can leverage the power of quantum computing within the constraints of current hardware. As quantum computers scale up in size and reliability, Classiq's software will continue to provide a flexible and accessible interface for harnessing their capabilities in the automotive industry.
The Road Ahead: Challenges and Opportunities for Quantum Computing in the EV Industry
While quantum computing offers immense potential for revolutionizing electric vehicle batteries and software, there are still significant challenges that need to be overcome before these benefits can be fully realized. One of the main hurdles is the development of fault-tolerant, large-scale quantum computers that can perform reliable, errorless computations on thousands of qubits. Current quantum hardware is still prone to errors and decoherence, which limit the depth and complexity of the algorithms that can be executed. Achieving fault-tolerance requires the implementation of quantum error correction codes, which themselves consume a large number of physical qubits to encode each logical qubit. Estimates suggest that millions of physical qubits may be necessary to simulate complex battery materials with chemical accuracy.
In the near-term, hybrid quantum-classical algorithms, such as variational quantum eigensolvers (VQE) and quantum approximate optimization algorithms (QAOA), offer a pragmatic approach to leveraging the power of quantum computers within their current limitations. These algorithms employ a quantum processor to execute a parameterized quantum circuit, while a classical optimizer is used to adjust the parameters based on the measurement outcomes. By iterating between the quantum and classical components, hybrid algorithms can find approximate solutions to complex optimization problems, such as molecular ground states or optimal control policies. However, the performance of these algorithms is still limited by the noise and scale of current quantum hardware.
As quantum technologies continue to advance, it is crucial to establish a realistic timeline for when quantum advantage might be achieved in the domain of electric vehicles. In the next 5 years, it is likely that quantum computers will be used primarily for small-scale optimization problems and proof-of-concept demonstrations of QML. Significant progress in battery materials discovery and optimization is expected within a 10-year horizon, as quantum simulators with hundreds of qubits become available. However, fully fault-tolerant quantum computers capable of performing accurate, large-scale simulations of entire battery systems may still be 15-20 years away.
To prepare for this quantum future, automotive companies gradually tart engaging with the quantum ecosystem and building in-house expertise. Partnerships between car manufacturers, battery suppliers, and quantum technology companies will be essential to guide the development of quantum algorithms and hardware tailored to the specific needs of the electric vehicle industry. Equally important is the cultivation of a quantum-ready workforce, with cross-disciplinary skills in chemistry, materials science, computer science, and quantum information theory. By investing in these areas now, the automotive sector can position itself to harness the transformative power of quantum computing as it becomes a practical reality in the coming decades.
About "The Qubit Guy's Podcast"
Hosted by The Qubit Guy (Yuval Boger, our Chief Marketing Officer), the podcast hosts thought leaders in quantum computing to discuss business and technical questions that impact the quantum computing ecosystem. Our guests provide interesting insights about quantum computer software and algorithm, quantum computer hardware, key applications for quantum computing, market studies of the quantum industry and more.
If you would like to suggest a guest for the podcast, please contact us.